Edge Intelligence or Edge AI strikes AI computing from the cloud to edge gadgets, the place information is generated. This can be a key to constructing distributed and scalable AI methods in resource-intensive purposes similar to Pc Imaginative and prescient.
On this article, we talk about the next matters:
- What’s Edge Computing, and why do we’d like it?
- What’s Edge Intelligence or Edge AI?
- Shifting Deep Studying Purposes to the Edge
- On-Gadget AI and Inference on the Edge
- Edge Intelligence allows AI democratization
Edge Computing Traits
With the breakthroughs in deep studying, current years have witnessed a booming of synthetic intelligence (AI) purposes and companies. Pushed by the fast advances in cell computing and the Synthetic Intelligence of Issues (AIoT), billions of cell and IoT gadgets are linked to the Web, producing zillions of bytes of information on the community edge.
Accelerated by the success of AI and IoT applied sciences, there’s an pressing must push the AI frontiers to the community edge to completely unleash the potential of huge information. To understand this pattern, Edge Computing is a promising idea to assist computation-intensive AI purposes on edge gadgets.
Edge Intelligence or Edge AI is a mixture of AI and Edge Computing; it allows the deployment of machine studying algorithms to the sting gadget the place the information is generated. Edge Intelligence has the potential to offer synthetic intelligence for each individual and each group at anywhere.
What’s Edge Computing
Edge Computing is the idea of capturing, storing, processing, and analyzing information nearer to the placement the place it’s wanted to enhance response occasions and save bandwidth. Therefore, edge computing is a distributed computing framework that brings purposes nearer to information sources similar to IoT gadgets, native finish gadgets, or edge servers.
The rationale of edge computing is that computing ought to occur within the proximity of information sources. Subsequently, we envision that edge computing might have as huge an affect on our society as we have now witnessed with cloud computing.
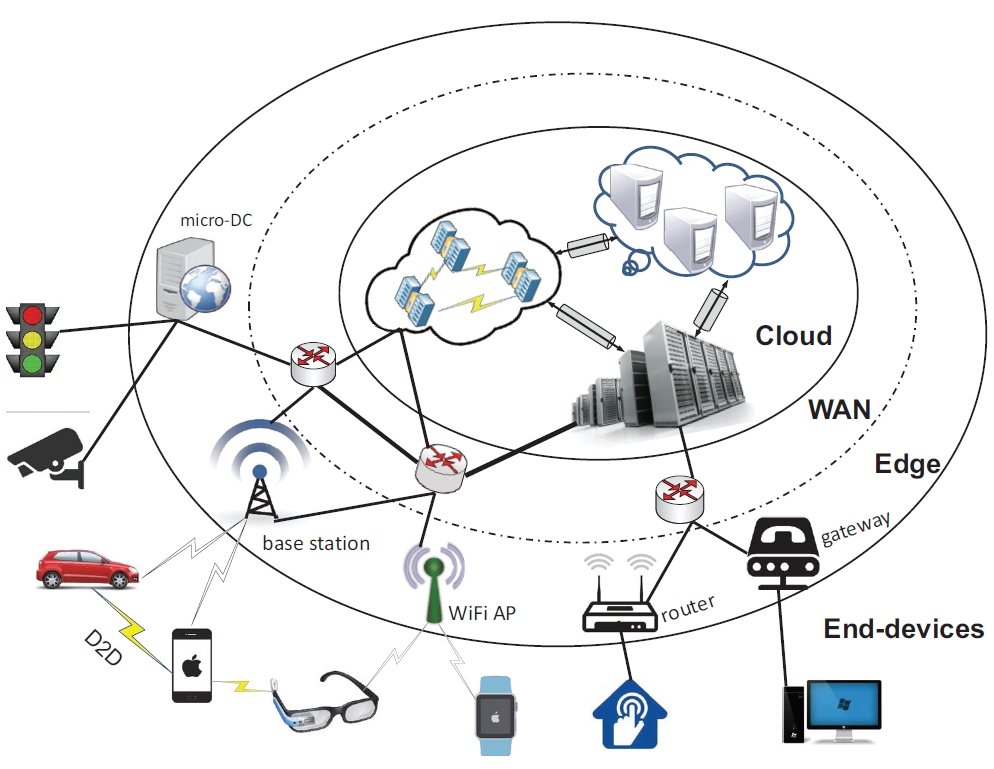
Why We Want Edge Intelligence
Information Is Generated On the Community Edge
As a key driver that reinforces AI growth, huge information has not too long ago gone via a radical shift of information sources from mega-scale cloud information facilities to more and more widespread finish gadgets, similar to cell, edge, and IoT gadgets. Historically, huge information, similar to on-line purchasing information, social media content material, and enterprise informatics, have been primarily born and saved at mega-scale information facilities. Nonetheless, with the emergence of cell computing and IoT, the pattern is reversing now.
Immediately, giant numbers of sensors and sensible gadgets generate huge quantities of information, and ever-increasing computing energy is driving the core of computations and companies from the cloud to the sting of the community. Immediately, over 50 billion IoT gadgets are linked to the Web, and the IDC forecasts that, by 2025, 80 billion IoT gadgets and sensors will probably be on-line.
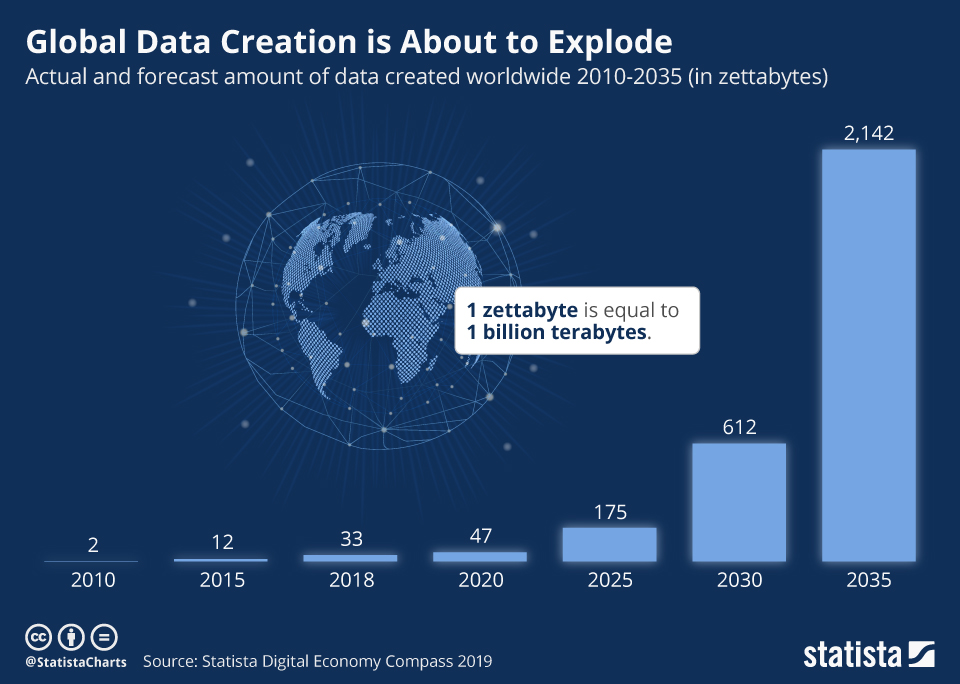
Cisco’s World Cloud Index estimates that just about 850 Zettabytes (ZB) of information collected will probably be generated every year outdoors the cloud by 2021, whereas international information heart visitors was projected to be solely 20.6 ZB. This means that the sources of information are reworking – from large-scale cloud information facilities to an more and more wide selection of edge gadgets. In the meantime, cloud computing is steadily unable to handle these massively distributed computing energy and analyze their information:
- Assets: Shifting an amazing quantity of collected information throughout the wide-area community (WAN) poses severe challenges to community capability and the computing energy of cloud computing infrastructures.
- Latency: For cloud-based computing, the transmission delay might be prohibitively excessive. Many new kinds of purposes have difficult delay necessities that the cloud would have problem assembly constantly (e.g., cooperative autonomous driving).
Edge Computing Gives Information Processing On the Information Supply
Edge Computing is a paradigm to push cloud companies from the community core to the community edges. The aim of Edge Computing is to host computation duties as shut as potential to the information sources and end-users.
Definitely, edge computing and cloud computing should not mutually unique. As an alternative, the sting enhances and extends the cloud. The principle benefits of mixing edge computing with cloud computing are the next:
- Spine community efficiency: Distributed edge computing nodes can deal with many computation duties with out exchanging the underlying information with the cloud. This enables for optimizing the visitors load of the community.
- Agile service response: Clever purposes deployed on the edge (AIoT) can considerably cut back the delay of information transmissions and enhance the response pace.
- Highly effective cloud backup: In conditions the place the sting can’t afford it, the cloud can present highly effective processing capabilities and large, scalable storage.
Information is more and more produced on the fringe of the community, and it might be extra environment friendly to additionally course of the information on the fringe of the community. Therefore, edge computing is a vital answer to interrupt the bottleneck of rising applied sciences based mostly on its benefits of lowering information transmission, bettering service latency, and easing cloud computing strain.
Edge Intelligence Combines AI and Edge Computing
Information Generated On the Edge Wants AI
The skyrocketing numbers and kinds of cell and IoT gadgets result in the technology of huge quantities of multi-modal information (audio, footage, video) of the gadget’s bodily environment which can be repeatedly sensed.
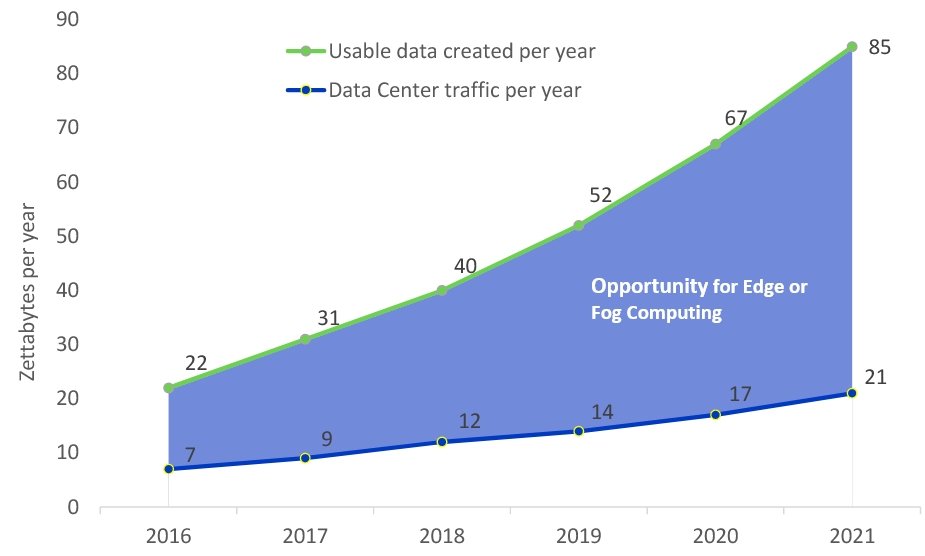
AI is functionally essential on account of its capacity to rapidly analyze big information volumes and extract insights from them for high-quality decision-making. Gartner forecasted that quickly, greater than 80% of enterprise IoT tasks will embrace an AI element.
Probably the most widespread AI methods, deep studying, brings the flexibility to determine patterns and detect anomalies within the information sensed by the sting gadget, for instance, inhabitants distribution, visitors circulate, humidity, temperature, strain, and air high quality.
The insights extracted from the sensed information are then fed to the real-time predictive decision-making purposes (e.g., security and safety, automation, visitors management, inspection) in response to the fast-changing environments, rising operational effectivity.
What’s Edge Intelligence and Edge ML
The mix of Edge Computing and AI has given rise to a brand new analysis space named “Edge Intelligence” or “Edge ML”. Edge Intelligence makes use of the widespread edge assets to energy AI purposes with out completely counting on the cloud. Whereas the time period Edge AI or Edge Intelligence is model new, practices on this path have begun early, with Microsoft constructing an edge-based prototype to assist cell voice command recognition in 2009.
Nonetheless, regardless of the early starting of exploration, there’s nonetheless no formal definition for edge intelligence. At present, most organizations and presses discuss with Edge Intelligence as “the paradigm of operating AI algorithms regionally on an finish gadget, with information (sensor information or indicators) which can be created on the gadget.”
Edge ML and Edge Intelligence are extensively regarded areas for analysis and business innovation. Because of the superiority and necessity of operating AI purposes on the sting, Edge AI has not too long ago acquired nice consideration.
The Gartner Hype Cycles names Edge Intelligence as an rising know-how that can attain a plateau of productiveness within the following 5 to 10 years. A number of main enterprises and know-how leaders, together with Google, Microsoft, IBM, and Intel, demonstrated the benefits of edge computing in bridging the final mile of AI. These efforts embrace a variety of AI purposes, similar to real-time video analytics, cognitive help, precision agriculture, sensible metropolis, sensible house, and industrial IoT.
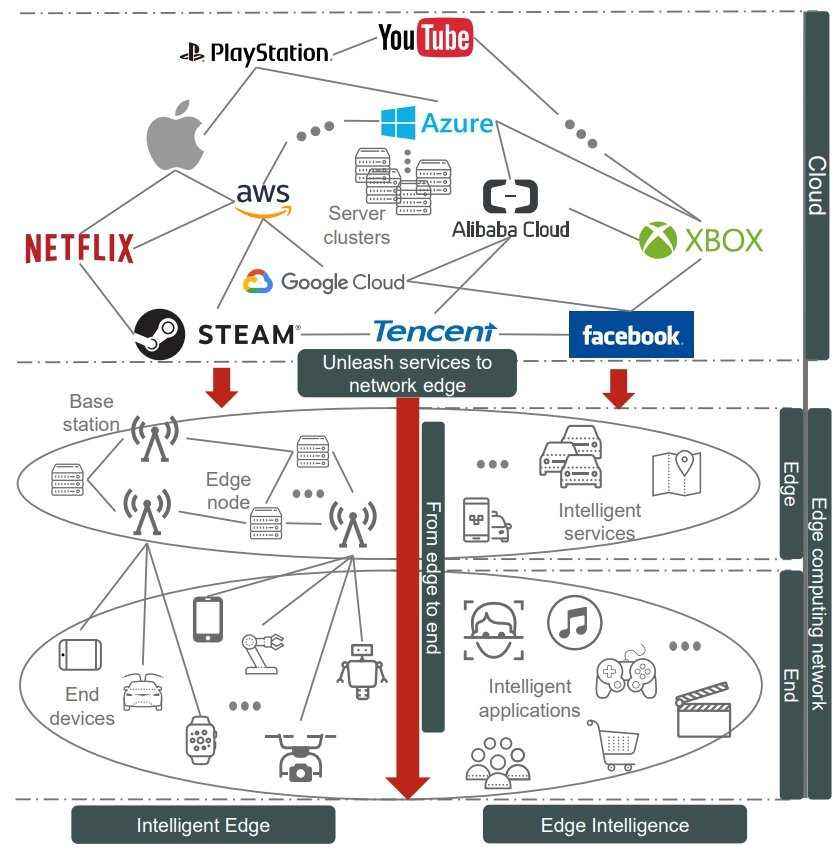
Cloud Is Not Sufficient to Energy Deep Studying Purposes
Synthetic Intelligence and deep learning-based intelligence companies and purposes have modified many features of individuals’s lives as a result of nice benefits of deep studying within the fields of Pc Imaginative and prescient (CV) and Pure Language Processing (NLP).
Nonetheless, on account of effectivity and latency points, the present cloud computing service structure isn’t sufficient to offer synthetic intelligence for each individual and each group at anywhere.
For a wider vary of software situations, similar to sensible factories and cities, face recognition, medical imaging, and so on., there are solely a restricted variety of clever companies supplied as a result of following elements:
- Value: The coaching and inference of deep studying fashions within the cloud require gadgets or customers to transmit huge quantities of information to the cloud. This consumes an immense quantity of community bandwidth.
- Latency: The delay in accessing cloud companies is mostly not assured and may not be quick sufficient for a lot of time-critical purposes.
- Reliability: Most cloud computing purposes depend upon wi-fi communications and spine networks for connecting customers to companies. For a lot of industrial situations, clever companies should be extremely dependable, even when community connections are misplaced.
- Privateness: Deep Studying usually includes an enormous huge quantity of personal data. AI privateness points are essential to areas similar to sensible houses, sensible manufacturing, autonomous autos, and sensible cities. In some circumstances, even the transmission of delicate information is probably not potential.
For the reason that edge is nearer to customers than the cloud, edge computing is predicted to unravel many of those points.
Benefits of Shifting Deep Studying to the Edge
The fusion of AI and edge computing is pure since there’s a clear intersection between them. Information generated on the community edge is determined by AI to completely unlock its full potential. And edge computing is ready to prosper with richer information and software situations.
Edge intelligence is predicted to push deep studying computations from the cloud to the sting as a lot as potential. This allows the event of assorted distributed, low-latency, and dependable, clever companies.
The benefits of deploying deep studying to the sting embrace:
- Low-Latency: Deep Studying companies are deployed near the requesting customers. This considerably reduces the latency and value of sending information to the cloud for processing.
- Privateness Preservation: Privateness is enhanced for the reason that uncooked information required for deep studying companies is saved regionally on edge gadgets or consumer gadgets themselves as a substitute of the cloud.
- Elevated Reliability: Decentralized and hierarchical computing structure supplies extra dependable deep studying computation.
- Scalable Deep Studying: With richer information and software situations, edge computing can promote the widespread software of deep studying throughout industries and drive AI adoption.
- Commercialization: Diversified and invaluable deep studying companies broaden the business worth of edge computing and speed up its deployment and progress.
Unleashing deep studying companies utilizing assets on the community edge, close to the information sources, has emerged as a fascinating answer. Subsequently, edge intelligence goals to facilitate the deployment of deep studying companies utilizing edge computing.
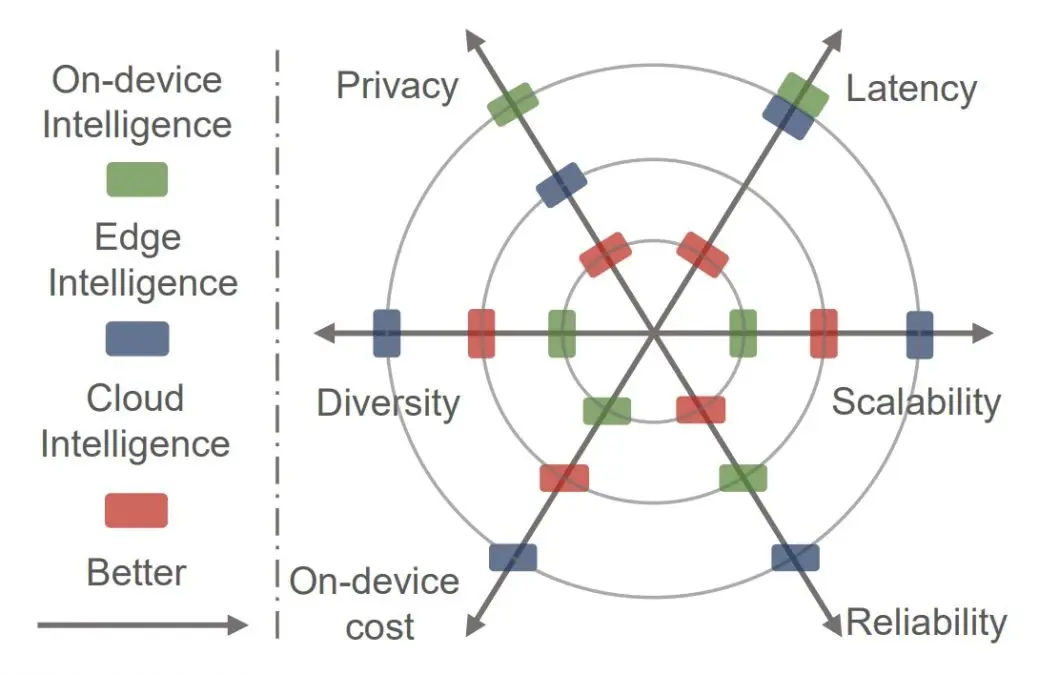
Edge Computing Is the Key Infrastructure for AI Democratization
AI applied sciences have witnessed nice success in lots of digital services or products in our day by day lives (e-commerce, service suggestions, video surveillance, sensible house gadgets, and so on.). Additionally, AI is a key driving power behind rising progressive frontiers, similar to self-driving vehicles, clever finance, most cancers analysis, sensible cities, clever transportation, and medical discovery.
Based mostly on these examples, leaders in AI push to allow a richer set of deep studying purposes and push the boundaries of what’s potential. Therefore, AI democratization or ubiquitous AI is a aim declared by main IT corporations, with the imaginative and prescient of “making AI for each individual and each group all over the place.”
Subsequently, AI ought to transfer “nearer” to the folks, information, and finish gadgets. Clearly, edge computing is extra competent than cloud computing in attaining this aim:
- In comparison with cloud information facilities, edge servers are in nearer proximity to folks, information sources, and gadgets.
- In comparison with cloud computing, edge computing is extra inexpensive and accessible.
- Edge computing has the potential to offer extra various software situations of AI than cloud computing.
As a consequence of these benefits, edge computing is of course a key enabler for ubiquitous AI.
Multi-Entry Edge Computing (MEC)
What’s Multi-Entry Edge Computing?
Multi-access Edge Computing (MEC), also called Cellular Edge Computing, is a key know-how that permits cell community operators to leverage edge-cloud advantages utilizing their 5G networks.
Following the idea of edge computing, MEC is situated close to the linked gadgets and end-users and allows extraordinarily low latency and excessive bandwidth whereas at all times enabling purposes to leverage cloud capabilities as essential.
MEC to leverage 5G and AI
Lately, the MEC paradigm has attracted nice curiosity from each academia and business researchers. Because the world turns into extra linked, 5G guarantees vital advances in computing, storage, and community efficiency in several use circumstances. That is how 5G, together with AI, has the potential to energy large-scale AI purposes, for instance, in agriculture or logistics.
The brand new technology of AI purposes produces an enormous quantity of information and requires quite a lot of companies, accelerating the necessity for excessive community capabilities when it comes to excessive bandwidth, ultra-low latency, and useful resource consumption for compute-intensive duties similar to laptop imaginative and prescient.
Therefore, telecommunication suppliers are progressively trending towards Multi-access Edge Computing (MEC) know-how to enhance the offered companies and considerably improve cost-efficiency. Because of this, telecommunication and IT ecosystems, together with infrastructure and repair suppliers, are in full technological transformation.
How does Multi-Entry Edge Computing work?
MEC consists of shifting the totally different assets from distant centralized cloud infrastructure to edge infrastructure nearer to the place the information is produced. As an alternative of offloading all the information to be computed within the cloud, edge networks act as mini information facilities that analyze, course of, and retailer the information.
Because of this, MEC reduces latency and facilitates high-bandwidth purposes with real-time efficiency. This makes it potential to implement Edge-to-Cloud methods with out the necessity to set up bodily edge gadgets and servers.
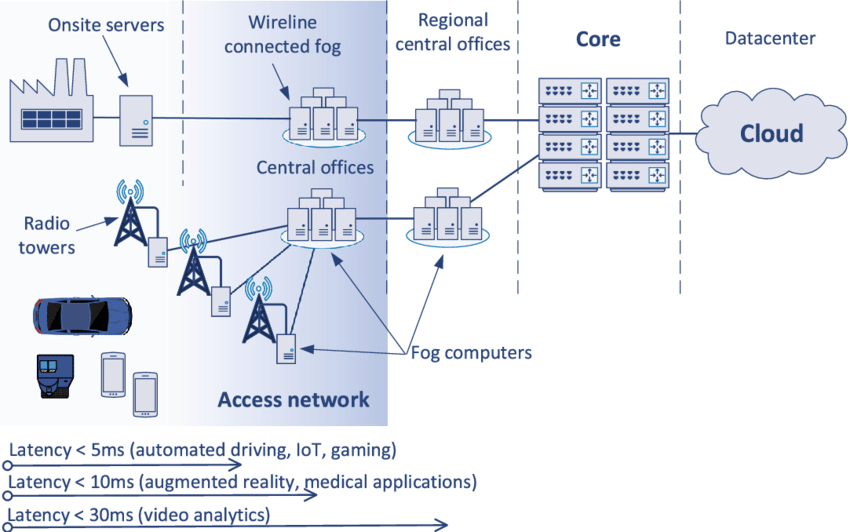
Pc Imaginative and prescient and MEC
Combining state-of-the-art laptop imaginative and prescient algorithms similar to Deep Studying algorithms and MEC supplies new benefits for large-scale, onsite visible computing purposes. In Edge AI use circumstances, MEC leverages virtualization to exchange bodily edge gadgets and servers with digital gadgets to course of heavy workloads similar to video streams despatched via a 5G connection.
At viso.ai, we offer an end-to-end laptop imaginative and prescient platform to construct, deploy, and function AI imaginative and prescient purposes. Viso Suite supplies full edge gadget administration to securely roll out purposes with automated deployment capabilities and distant troubleshooting.
The sting-to-cloud structure of Viso helps seamlessly enrolling not solely bodily but in addition digital edge gadgets. In collaboration with Intel engineers, we’ve built-in the virtualization capabilities to seamlessly enroll digital edge gadgets on MEC servers.
Because of this, organizations can construct and ship laptop imaginative and prescient purposes utilizing the low-latency and scalable Multi-access Edge Computing infrastructure. For instance, in Good Metropolis, the MEC of a cell community supplier can be utilized to attach IP cameras all through town and run a number of real-time AI video analytics purposes.
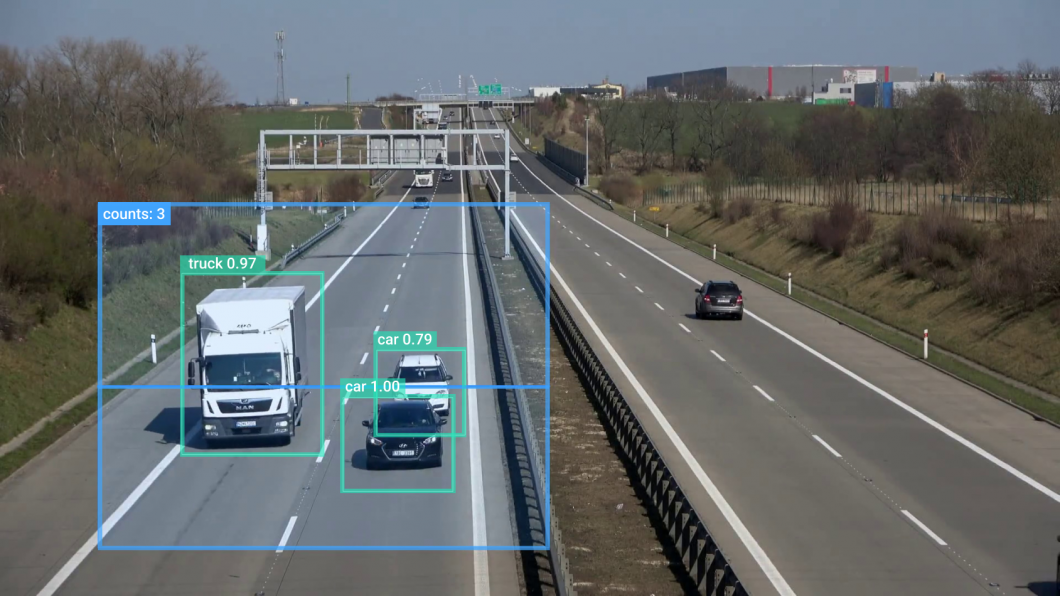
Deployment of Machine Studying Algorithms on the Community Edge
The unprecedented quantity of information, along with the current breakthroughs in synthetic intelligence (AI), allows the usage of deep studying know-how. Edge Intelligence allows the deployment of machine-learning algorithms on the community edge.
The important thing motivation for pushing studying in direction of the sting is to permit fast entry to the big real-time information generated by the sting gadgets for quick AI-model coaching and inferencing, which in flip endows on the gadgets with human-like intelligence to answer real-time occasions.
On-device analytics run AI purposes on the gadget to course of the gathered information regionally. As a result of many AI purposes require excessive computational energy that enormously outweighs the capability of resource- and energy-constrained edge gadgets. Subsequently, the dearth of efficiency and power effectivity are frequent challenges of Edge AI.
Completely different Ranges of Edge Intelligence
Most ideas of Edge Intelligence usually deal with the inference section (operating the AI mannequin) and assume that the coaching of the AI mannequin is carried out in cloud information facilities, principally as a result of excessive useful resource consumption of the coaching section.
Nonetheless, the total scope of Edge Intelligence absolutely exploits obtainable information and assets throughout the hierarchy of finish gadgets, edge nodes, and cloud information facilities to optimize the general efficiency of coaching and inferencing a Deep Neural Community mannequin.
Subsequently, Edge Intelligence doesn’t essentially require the deep studying mannequin to be absolutely educated or inference on the edge. Therefore, there are cloud-edge situations that contain information offloading and co-training.
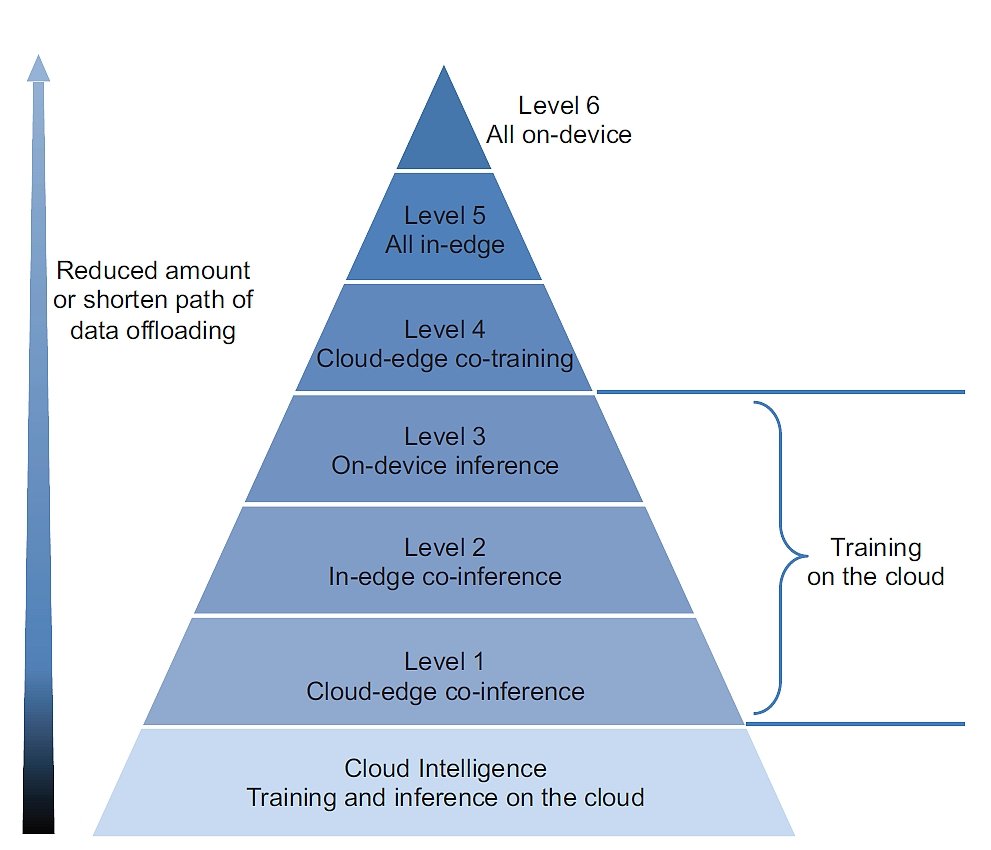
There is no such thing as a “greatest degree” usually as a result of the optimum setting of Edge Intelligence is application-dependent and is decided by collectively contemplating a number of standards similar to latency, privateness, power effectivity, useful resource value, and bandwidth value.
- Cloud Intelligence is the coaching and inferencing of AI fashions absolutely within the cloud.
- On-device Inference consists of AI mannequin coaching within the cloud, whereas AI inferencing is utilized in a totally native on-device method. On-device inference signifies that no information could be offloaded.
- All On-Gadget is performing each coaching and inferencing of AI fashions absolutely on-device.
By shifting duties in direction of the sting, transmission latency of information offloading decreases, information privateness will increase and cloud useful resource and bandwidth prices are lowered. Nonetheless, that is achieved at the price of elevated power consumption and computational latency on the edge.
On-device Inference is presently a promising method for numerous on-device AI purposes which have been confirmed to be optimally balanced for a lot of use circumstances. On-device mannequin coaching is the inspiration of Federated Studying.
Deep Studying On-Gadget Inference on the Edge
AI fashions, extra particularly Deep Neural Networks (DNNs), require larger-scale datasets to additional enhance their accuracy. This means that computation prices dramatically improve, because the excellent efficiency of Deep Studying fashions requires high-level {hardware}. Because of this, it’s tough to deploy them to the sting, which comes with useful resource constraints.
Subsequently, large-scale deep studying fashions are usually deployed within the cloud whereas finish gadgets simply ship enter information to the cloud after which look forward to the deep studying inference outcomes. Nonetheless, the cloud-only inference limits the ever present use of deep studying companies:
- Inference Latency. Particularly, it can’t assure the delay requirement of real-time purposes, similar to real-time detection with strict latency calls for.
- Privateness. Information security and privateness safety are necessary limitations of cloud-based inference methods.
To handle these challenges, deep studying companies are inclined to resort to edge computing. Subsequently, deep studying fashions need to be personalized to suit the resource-constrained edge. In the meantime, deep studying purposes must be fastidiously optimized to steadiness the trade-off between inference accuracy and execution latency.
What’s Subsequent for Edge Intelligence and Edge Computing?
With the emergence of each AI and IoT comes the necessity to push the AI frontier from the cloud to the sting gadget. Edge computing has been a well known answer to assist computation-intensive AI and laptop imaginative and prescient purposes in resource-constrained environments.
Viso Suite makes it potential for enterprises to combine laptop imaginative and prescient and edge AI into their enterprise workflows. Really end-to-end, Viso Suite removes the necessity for level options, that means that groups can handle the purposes in a unified infrastructure. Be taught extra by reserving a demo with our crew.
Clever Edge, additionally known as Edge AI, is a novel paradigm of bringing edge computing and AI collectively to energy ubiquitous AI purposes for organizations throughout industries. We suggest you learn the next articles that cowl associated matters:
References: