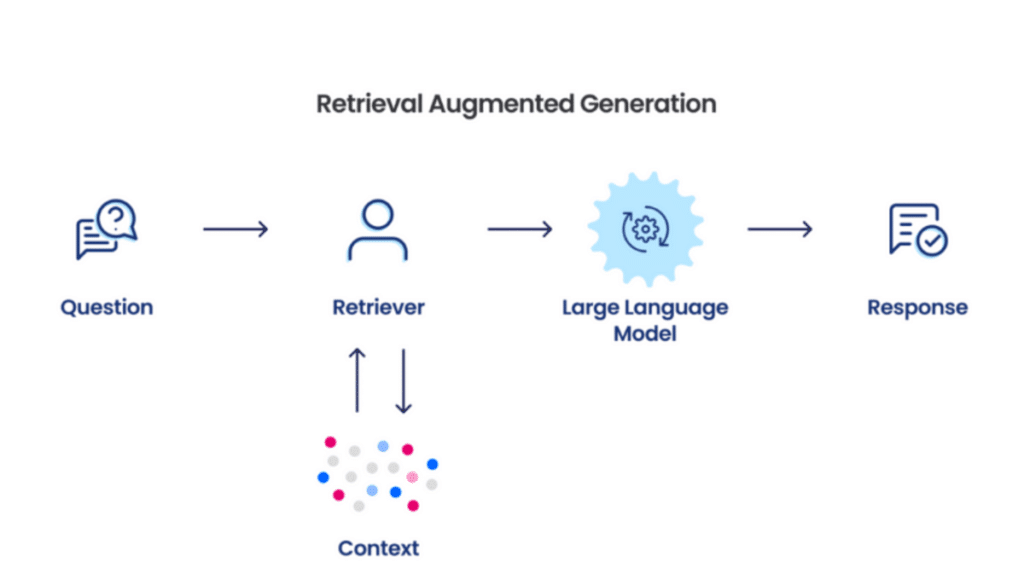
Unstructured knowledge, encompassing codecs comparable to textual content, pictures, audio, and video, makes up a majority of the info out there within the digital age, typically missing a predefined knowledge mannequin or not simply becoming into relational tables.
As companies and entities try and extract beneficial insights from copious quantities of unstructured data, applied sciences like Retrieval-Augmented Era (RAG) have gained prominence. This text elucidates the intersection of unstructured knowledge with RAG know-how, delineating its capabilities and challenges in up to date data-driven eventualities.
Understanding Unstructured Information
Unstructured knowledge refers to any knowledge that doesn’t have a recognizable construction or just isn’t organized in a pre-defined method. This could embrace:
- Textual content: Emails, books, articles, and social media posts.
- Photos: Images, satellite tv for pc pictures, and medical scans.
- Audio: Voice recordings, music recordsdata, and spoken content material.
- Video: Motion pictures, surveillance footage, and private movies.
These knowledge kinds are primarily generated from diversified sources like social media platforms, enterprise transactions, multimedia content material, and communication channels throughout numerous industries comparable to healthcare, finance, media, and leisure. The richness of this knowledge supplies a fertile floor for deriving insights but presents vital challenges by way of processing and evaluation as a consequence of its measurement and complexity.
The Significance of RAG in Dealing with Unstructured Information
RAG, or Retrieval-Augmented Era, is a hybrid mannequin combining the advantages of retrieval-based and generative AI programs. The mannequin enhances response accuracy and relevance by retrieving data from an unlimited database of data earlier than producing content material. This two-step strategy permits the system to contextualize responses higher, making it exceptionally useful for dealing with the nuances of unstructured knowledge.
Technological Framework of RAG
The operational framework of a RAG system entails a number of subtle elements working synchronously:
- Immediate Submission: A person question is obtained as an enter immediate to the RAG system.
- Information Retrieval: The system queries a vector database, retrieving related paperwork or knowledge snippets based mostly on semantic similarity to the enter.
- Processing by LLM: The retrieved content material, together with the unique immediate, is fed into a big language mannequin (LLM) like GPT (Generative Pre-trained Transformer). The LLM synthesizes the data to formulate a coherent and contextually acceptable response.
- Response Supply: The response generated by the LLM is returned to the person, finishing the interplay cycle.
For an in depth information on establishing such a RAG pipeline, consult with this put up: How to Build a RAG Pipeline.
Energy of RAG in Using Unstructured Information
The mixing of RAG programs successfully addresses many challenges related to unstructured knowledge. By leveraging state-of-the-art machine studying fashions for each retrieval and era, RAG can considerably improve the precision of knowledge processing. The accuracy of content material retrieval is especially important because it ensures the relevance of the generated outputs, thereby lowering the noise and enhancing the standard of knowledge introduced to customers.
Challenges in Managing Unstructured Information with RAG
Regardless of its benefits, the deployment of RAG programs entails a number of challenges:
- Scalability: Dealing with huge quantities of knowledge requires immense computational assets and complex scaling methods.
- API Overload: Frequent and sophisticated queries can overwhelm the system, resulting in delays or failures in knowledge retrieval.
- Information Relevance: Making certain that the retrieved data stays related and up-to-date is an ongoing problem, notably with constantly evolving knowledge units.
Moral and Safety Issues
The dealing with of delicate and personal data inside unstructured knowledge swimming pools requires diligent moral concerns and strong safety protocols to stop knowledge breaches and guarantee person privateness.
Future Traits in RAG and Unstructured Information
The continued developments in AI and machine studying are poised to additional refine the capabilities of RAG programs. With the continual enhance in knowledge manufacturing, adaptive and extra subtle RAG fashions are anticipated to emerge, catering to numerous sectors and functions.